The Critical Role of Analyzing Historical Data for Future Insights
Data is the very lifeblood running through the enterprise, fueling every aspect of a modern business: from the understanding of customer behavior to streamlining enterprise operations. The catch? It’s not the volume of data that matters but how you use it. The key to actionable insights is in historical data; it is an ample treasure of previous trends that can forecast future outcomes.
Organizations driven by data have experienced a remarkable 63% increase in the speed of their processes’ productivity. On the other hand, data-driven organizations have triple the likelihood of reporting a significant increase in the quality of their decision-making.
Why? Simply because historical data allows you to rise above guesswork and make data-based predictions accurately for the first time. Imagine predicting churn in your customer base or predicting peak sales cycles to optimize the inventory. And that’s the power of analyzing historical data.
In the first part of our series, we delved into how a leading retail enterprise gauged the need for AI in fine-tuning its service operations. Now, in part two, we go on to the next important step: analyzing historical data to make precise predictions. Proper data analysis is necessary to leverage AI effectively, and we detail how the retail enterprise went about this. Let us call our enterprise RetailUno to make the process clear and easy to understand.
How to Analyze Historical Data for Right Predictions?
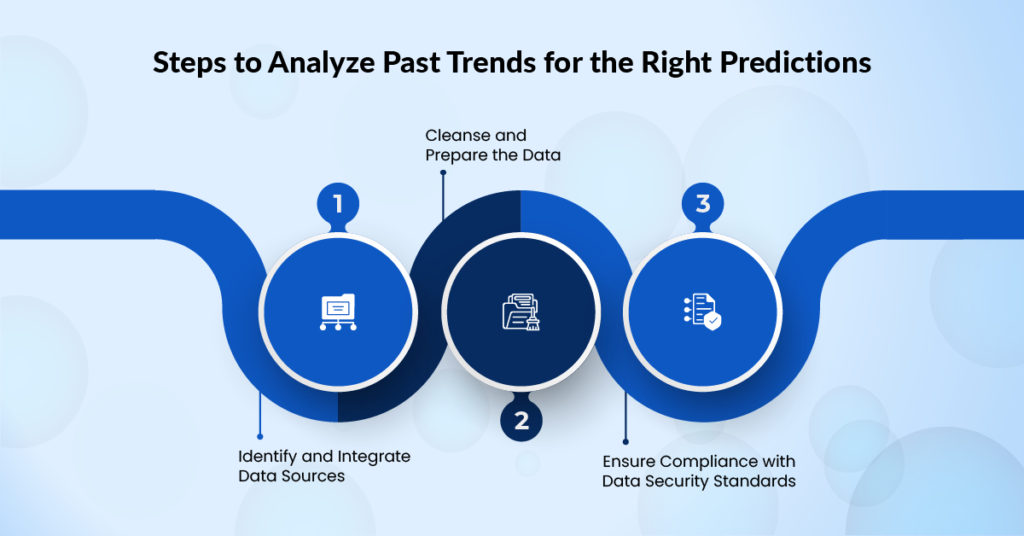
For AI to deliver valuable insights, it must be fed with high-quality data. The retail enterprise followed a structured approach to ensure their data was ready for AI analysis.
1. Identify and Integrate Data Sources
The first step in the analysis process was identifying and integrating all relevant data sources based on their defined objectives. RetailUno aimed to improve customer service, minimize downtime, and retain valuable customers. Therefore, they focused on collecting data that would support these goals.
- Customer Service Records: They gathered data from customer interactions, support tickets, and feedback forms. This data helped them understand common issues and customer satisfaction levels.
- Equipment Performance Logs: Data from equipment sensors and maintenance logs were crucial for predicting potential failures and maintenance needs.
- Sales and Marketing Data: Information on customer purchase history, engagement metrics, and campaign results provided insights into customer behavior and preferences.
By integrating these diverse data sources into a unified platform, RetailUno ensured that their AI system had a comprehensive view of their operations.
2. Cleanse and Prepare the Data
Once the data sources were identified and integrated, the next step was to cleanse and prepare the data for analysis.
- Data Cleansing: This involved removing inaccuracies, duplicates, and inconsistencies from the data. For instance, they corrected misspelled customer names, standardized date formats, and eliminated redundant records.
- Data Normalization: They normalized the data to ensure consistency across different sources. This included standardizing units of measurement, aligning data formats, and resolving any discrepancies.
- Data Enrichment: They also enriched their data by filling in missing values and adding relevant context. For example, they supplemented customer records with additional demographic information and updated equipment logs with recent performance metrics.
With the detailed process of data cleansing & preparation, the enterprise enhanced the reliability of its data, making it more suitable for AI analysis.
3. Ensure Compliance with Data Security Standards
Maintaining data security and privacy is paramount, especially when dealing with sensitive customer information. The retail business took several measures to ensure compliance with data security standards.
- Adhering to Regulations: RetailUno’s adherence to industry standards and regulations included GDPR, CCPA, and other data protection laws. This involved implementing data encryption, secure access controls, and regular audits.
- Data Governance Policies: RetailUno established robust data governance policies to manage data access, usage, and retention. This included defining roles and responsibilities, setting data access permissions, and implementing data retention schedules.
- Continuous Monitoring: The enterprise continuously monitored their data systems for any security breaches or vulnerabilities. They also conducted regular security assessments and updated their security protocols as needed.
By prioritizing data security, the company safeguarded its customers’ data & built trust in its AI initiatives.
Read More: An Overview of 3A’s of Right Salesforce Einstein AI Implementation
Conclusion
We explored how RetailUno used its historical data to make accurate future predictions. Having identified and integrated all the relevant data at the proper time, cleaned the data, prepared it, and ensured it conformed to the data security standards, they laid a strong foundation for using Salesforce Einstein AI effectively. Stay tuned for the last part of this series, where we’ll continue discussing how RetailUno acted upon the predictive recommendations of their AI system to run their operations better and achieve business outcomes.
Stay tuned for Part 3, where we will delve into how the enterprise used predictive insights to segment customers and used personalized outreach strategies for proactive maintenance.
Author
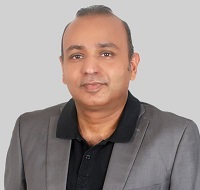
Global COO, AblyPro
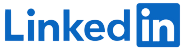
For 20 years, Neeraj has worked alongside a multitalented team to help associations and nonprofits drive digital transformation within their organization, enabling them to be more innovative, agile, and donor/member-centric. As AblyPro’s Global COO, he leads an internal task force that shares lessons learned, best practices, and practical applications that specifically relate to associations and nonprofits. With 300+ developers by his side, Neeraj provides clients with the resources and capacity to power up their teams.