A few years ago, the service industry struggled to improve operations. However, today AI is increasingly helping firms turn data into useful insights, streamline operations, and innovate. But everything starts with the first step of understanding the problem statement and well-defined goals to realize AI’s full potential. These strategic steps lead to improved ROI, increased productivity, and optimized service delivery. However, achieving this requires a comprehensive evaluation encompassing business challenges, clear goal-setting, stakeholder engagement, and the identification of specific use cases for ongoing progress.
This blog post will guide you through a three-step process to meticulously identify your problem statement and define objectives, ensuring your AI journey is both effective and aligned with your enterprise’s vision.
How to Define Problem Statement and Objectives in 3 Simple Steps
Step 1: Identifying Business Challenges
Before diving into AI implementation, organizations must pinpoint specific business challenges or opportunities where AI can add significant value. Consider the following:
- Business Context: Understand the context in which your organization operates. What pain points or inefficiencies, exist? Are there areas where manual processes can be automated or where data-driven insights can drive better decision-making?
- Stakeholder Input: Engage with stakeholders across different functions—operations, marketing, finance, customer service, etc. Gather insights on pain points, bottlenecks, and areas for improvement.
- Data Exploration: Assess the current data to target trends, irregularities, and growth opportunities. This technique easily identifies repeated tasks, resource-intensive operations, and decision-making barriers.
- Comparison Analysis: Conduct a comparison analysis of your organization’s performance to industrial benchmarks. Are there gaps that AI could address? Such as reducing customer churn percentage, improving supply chain efficiency, or enhancing product recommendations.
Step 2: Defining Clear Objectives
Once you’ve identified the problem areas, it’s essential to define clear objectives for your AI implementation. Consider the following:
- Quantifiable Outcomes: Objectives should be measurable, such as cost reduction through automation, accuracy improvement in predictions, and efficiency gains in processes.
- Specific Metrics: Success criteria should be defined using specific metrics like percentage reduction in processing time, increase in customer satisfaction scores, or higher conversion rates.
- Alignment with Business Goals: AI objectives must align with the broader business goals, contributing directly to strategic outcomes like revenue growth, customer retention, or operational efficiency.
Step 3: Choosing Relevant Use Cases
Not all problems require AI solutions. Choose use cases that align with strategic priorities and have a significant impact. Consider the following factors:
- Business Operations: Get a clear picture of what directly affects revenue, cost savings, or customer experience. For example, personalized recommendations can boost sales, and fraud detection helps prevent financial losses.
- Feasibility: Evaluate the feasibility of implementing AI for each specific use case. Consider data availability, technical requirements, and organizational readiness.
- Urgency: Address pressing challenges first. If a problem is causing immediate harm or hindering growth, prioritize it over less critical issues.
Now that you’ve defined your problem statement and objectives in three simple steps, it’s important to be aware of potential pitfalls. In the next section, we’ll highlight three common pitfalls to avoid, ensuring your objectives stay clear and achievable.
3 Common Pitfalls in Defining Objectives and How to Resolve Them
Unclear Objectives: Having objectives that are not clear enough or that are too broad can result in scope creep and weakened efforts. Make certain that the objectives are both obvious and detailed.
Solution: To resolve these common pitfalls, start by ensuring your objectives are clear and detailed. Break down broad goals into specific, actionable steps to prevent scope creep and maintain focused efforts.
Less Stakeholder Engagement: It involves less or zero participation of stakeholders within a company or project. When stakeholders aren’t engaged, it can lead to resistance to change & less support for critical initiatives, mainly in the context of AI projects within enterprises.
Solution: For this issue, actively engage stakeholders throughout the project. Involve them in the planning stages, gather their input, and keep them informed to gain their support and reduce resistance to change.
Overlooking Data Requirements: Disregarding both the quality and availability of data can lead to frequent project delays and increased costs.
Solution: You can address data requirements early in the process. Assess the quality and availability of your data and make necessary improvements or adjustments to avoid project delays and additional costs.
The Bottom Line
At last, when it comes to implementing AI effectively, it all starts with understanding the problem at hand and defining clear goals. Think of it like setting coordinates on a map before embarking on a journey. Next, align those AI initiatives with your business needs. Think of it like puzzle assembling: the better the pieces fit together, the smoother the process. However, keep in mind that AI isn’t a universal solution. Customizing your approach to your organization’s specific context is crucial. And to check your progress regularly to ensure that AI is delivering real value.
FAQs
AI is not necessary to overcome every business challenge, but it does help in enhancing business operations by automating mundane tasks, providing new revenue streams, and increasing efficiency. It allows for greater agility and predictability and offers insights from unstructured data.
Service operations often use manual methods and minimal data to make decisions. Whereas, an AI strategy leverages cutting-edge technologies like machine learning (or ML) & natural language processing (or NLP) to automate critical jobs-examining large data sets and making predictions. It leads to better client experiences, lower costs, and more efficient operations. Traditional approaches cannot provide real-time information and proactive service management like AI systems do.
Author
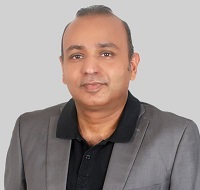
Global COO, AblyPro
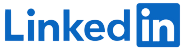
For 20 years, Neeraj has worked alongside a multitalented team to help associations and nonprofits drive digital transformation within their organization, enabling them to be more innovative, agile, and donor/member-centric. As AblyPro’s Global COO, he leads an internal task force that shares lessons learned, best practices, and practical applications that specifically relate to associations and nonprofits. With 300+ developers by his side, Neeraj provides clients with the resources and capacity to power up their teams.